Agentic AI is the next frontier in AI development, after generative AI.
Where generative AI creates responses, agentic AI goes beyond merely answering and performs complex actions necessary to reach expected user outcomes. It makes decisions and takes actions without much human intervention.
AI agents can delegate critical actions to humans. However, agentic AI automates the majority of decisions and operations before reaching critical actions. Most importantly, it does everything proactively without waiting for users to give a prompt. It makes the agent and customer experience seamless, moving them toward business objectives.
Where Do You Rank on the AI Maturity Curve?
Take the 5-minute assessment and get a personalized score and recommended next steps.
What Is Agentic AI?
Agentic AI is an advanced level of artificial intelligence automation that adapts to new data and learns on its own. It operates autonomously and executes tasks based on real-world data. The requirement for human intervention is minimal. Agentic AI plans, reasons, and adapts to optimize complex workflows for expected outcomes.
These autonomous agents can perceive their environment and make decisions with minimal human guidance. Traditional AI systems only act when prompted, but agentic AI plans and executes actions to achieve objectives, rather than simply generating output and stopping.
Overall, agentic AI doesn’t require a human prompt at every step to achieve its objective. It takes initiative, plans steps, and progresses toward the objective.
Key Characteristics of Agentic AI Systems
Below are some of the key characteristics of agentic AI systems.

Driven by objective
You provide the system, or it formulates specific objectives and works toward achieving them. It breaks down high-level goals into sub-tasks and plans action sequences. For example, you give an agent a goal to schedule a meeting. Agentic AI will find a time slot, invite attendees, book a meeting room, and alert all attendees sometime before the meeting begins. This is how it carries out multistep tasks.
Autonomous
These agents don’t need human approval at every step to accomplish an objective, either preset by a human or formulated by the agent on its own. However, this autonomy depends on how these agents are configured and can vary. Some agents might ask for confirmation before taking critical steps, while others simply reach the goal without virtual supervision.
For example, a trading agent can execute, sell or buy orders on the financial market without a person controlling it.
Interactive
Agentic AI interacts with the physical or virtual world and takes actions to affect it. Simply put, the agent responds to environmental changes and adapts accordingly. It allows these agents to operate in dynamic and unpredictable settings.
Adaptive
Agentic AI incorporates mechanisms to learn from experience. The more feedback you give, the better it adapts to new situations and environments. For example, a virtual assistant learns a user’s unique preferences after a few interactions, hyper-personalizing the user’s experience with the agent.
These agents aren’t stuck in a single hard-coded sequence, making them more flexible in handling unexpected events.

Persistent
Agentic AI operates over extended durations through multiple steps to achieve goals. This means that the agent can combine state and context over time, helping users achieve long-term objectives without needing much human intervention. Moreover, these agents are proactive rather than reactive. They take initiative while deciding what needs to be done and when. For example, an agent working on your email might draft a response for an urgent email.
This makes agentic AI not just any other tool in a user’s hand but a tool with a degree of self-directed agency.
How Does Agentic AI Work?
Agentic AI solves complex problems through a five-step process:
- Set a goal: Usually, a human gives agentic AI a set of goals to reach. Sometimes, the goal of agentic AI is derived from a higher objective. The goal can be as simple as “manage email inbox effectively.”
- Perceive: Various sources, such as sensors and databases, contribute to agentic AI’s data collection and processing. It extracts meaningful features and recognizes objects while identifying relevant environmental entities. This gives the agent context and situational awareness.
- Reason: Advanced AI models orchestrate reasoning, helping agentic AI understand specific tasks and coordinate specialized models for different visual processing. The agent uses retrieval-augmented generation (RAG) to access proprietary data sources and deliver accurate results. Agentic AI might also leverage a knowledge base for structured or unstructured data, neural networks for classification and prediction, and Belief-Desire-Intention (BDI) models to track its perception of the world.
- Act: After planning, agentic AI performs physical or digital actions in its environment. This action takes the agent toward the goal. The physical actions include activities like driving or navigation. On the other hand, digital action will involve sending an email, modifying records of a database, or making API calls.
- Learn: Agentic AI learns through feedback loops to refine and improve its performance over time. It can adapt and become more effective with feedback, helping businesses achieve better outcomes as it matures. It learns for reward signals or penalties and updates the model as new datasets enter the system.

Agentic AI in Sales and Customer Service
Below are some use cases of agentic AI on the sales side.
Sales use cases of agentic AI
Agentic AI can play a phenomenal role in sales, especially in lead qualification, sales outreach, dynamic pricing, and pipeline forecasting.
Automated lead qualification
Agentic AI ingests vast amounts of data from customer relationship management (CRM) software to get the context of a prospect’s behavior on a website. This connection with a CRM fuels the agent’s perception mechanism. It assesses the prospect’s interaction on the webpages and monitors their social interactions. These patterns tell whether a prospect is of high purchase intent. The agents continuously improve as they assess patterns and analyze how many such interactions convert into sales.
This makes it easier for the agent to prioritize high-intent prospects for human sales agents. Live agents can reach out to them methodically to build trust and take them toward conversion.

AI-powered sales outreach
With behavior data, agentic AI finds triggers where a potential buyer needs nudges to move toward the agent’s objective, i.e., sale or upsell. After identifying the trigger, the agent can draft emails or send relevant notifications to the buyer to automate follow-ups.
These notifications are automated follow-ups or trigger nurturing sequences to move the prospect toward signing a deal.
Dynamic pricing and upselling
Dynamic pricing requires real-time pricing adjustments based on market demand and competition. Agentic AI continuously scans competitors’ pricing, promotions, and availability to quote prices that ensure profits while still being competitive. These adjustments are implemented instantaneously based on external signals and market fluctuations.
This doesn’t mean that prices are reduced beyond the lowest feasible limit. If conditions are set, agentic AI won’t lower them below the set price. Even when it lowers the cost, it does so strategically. When the competitors run out of stock, the prices can be increased to recover any discounts given.

Agentic AI also considers customer interactions and purchase patterns to find the optimal price at which deals will likely go through. This maximizes conversions for individual customers. This is similar to the way travel booking platforms adjust pricing for customers based on their past bookings. The agent evaluates contextual data, such as holidays or time of day, to set prices. It adjusts them proactively to match anticipated demand fluctuations.
Similarly, the agent autonomously identifies upsell opportunities personalized for customers.
Pipeline forecasting and insights
Agentic AI analyzes historical data to study sales trends and identify bottlenecks in the process. Based on its analysis, it modifies workflows to guide users toward deal closures or sends recommendations to sales agents.
Agentic AI is connected to CRM, customer data platforms, and other business systems that consistently monitor customer behavior and agent activity. It uses data in these systems to run its analysis, delivering real-time insights to help sales representatives get a real picture of their pipeline. These insights allow realistic forecasting of the sales pipeline, keeping predicted numbers close to the actuals.
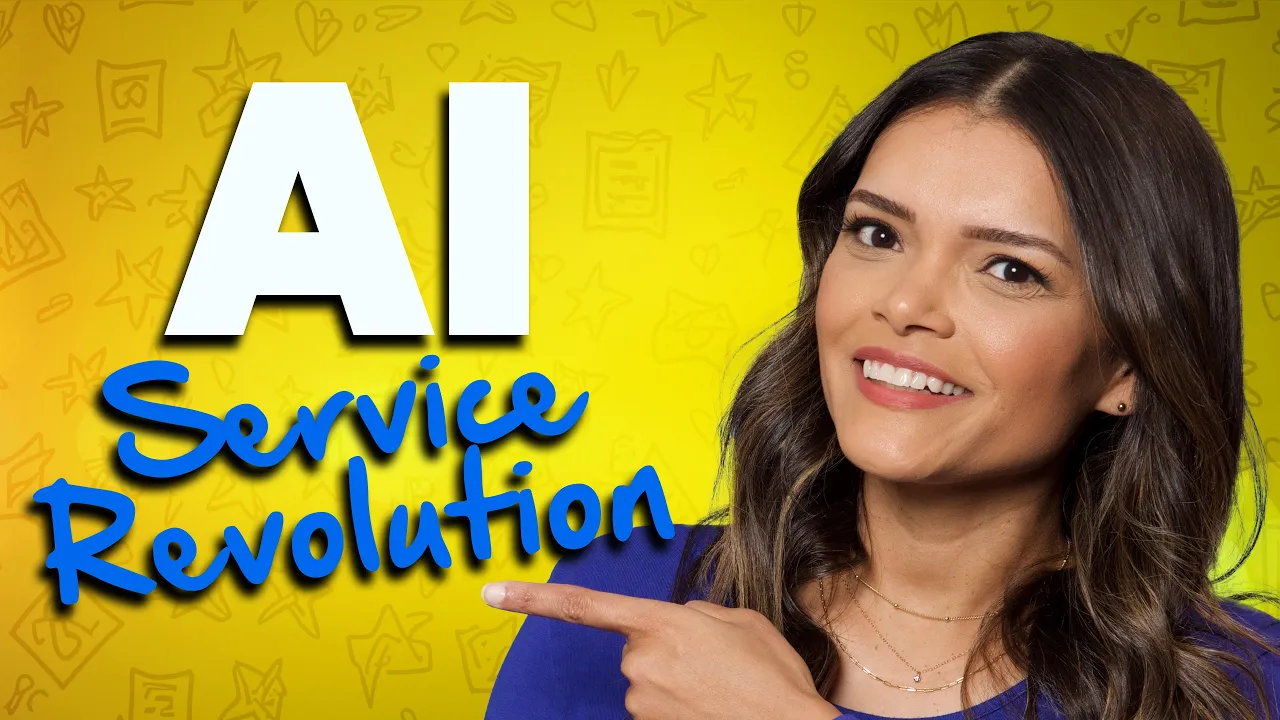
Customer service use cases of agentic AI
On the customer service front, agentic AI serves customers and the company in various ways.
Automated issue resolution
Agentic AI autonomously manages customer queries and takes them toward resolution on chat, voice, or email. This increases the first contact resolution rates at call centers while ensuring customers have a decent service experience.
These agents are available 24/7 to offer instantaneous support to deliver customer satisfaction whenever they reach out.
Nvidia’s article clearly states, “An AI agent for customer service, for instance, could operate beyond simple question-answering. Agentic systems could check a user’s outstanding balance and recommend which accounts could pay it off — all while waiting for the user to decide so it could complete the transaction accordingly when prompted.”
Proactive customer support
Agentic AI predicts potential service disruptions and alerts customers before issues escalate. The agents take the initiative to prevent dissatisfaction or churn. They are equipped with predictive analytics capabilities to identify patterns signaling potential customer issues.
Based on the actual usage data, these agents suggest personalized upgrades or alternative actions to avoid the issue in the future.
AI-guided human agent
Agents receive real-time insights when they’re in a conversation with a customer. This ensures the agent has everything they need to offer a quick resolution to the customer, drastically improving resolution speeds.
With agentic AI insights, customer service representatives can suggest the next course of action immediately. After successful interactions, the agent learns and improves its recommendations over time.

Omnichannel AI assistants
Modern contact centers have support systems integrated across email, social media, chat, and phone. They maintain a unified customer support channel to give buyers a consistent experience. Agentic AI can integrate with these systems through AI and deliver autonomous support across all communication channels. It retains context from previous interactions and offers a seamless experience to the customer without hurdles.
What You Need to Implement Agentic AI
Before implementing agentic AI, clearly define its use cases and the measurable business goals that you aspire to achieve with the technology. Only then should you move toward setting up the requirements.
Data infrastructure and integration
Agentic AI needs robust data collection, storage, and processing systems for real-time analysis and decision-making. Ensure your data warehouses or lakes give easy access for consolidating and cleaning existing data.
Integrate CRM software, ticketing systems, an enterprise resource planning (ERP) platform, and an analytics platform to support the AI agent in making informed decisions.
Workflow automation and APIs
Ensure that the tools in your tech stack support process automation triggered by artificial intelligence. AI-powered agents will leverage AI capabilities to perform various tasks across different platforms to reach their business objectives.
Make sure API-first platforms allow AI agents to trigger actions across third-party applications.
AI governance and compliance
AI can cause issues when it comes to compliance. Ensure you set strict guardrails to allow AI to follow GDPR, CCPA, and PCI-DSS compliance regulations. It’s best to have human oversight when making critical decisions.
On the cybersecurity front, relevant controls should be set up to avoid adversarial attacks and data breaches.
Continuous learning and optimization
Systems require ongoing monitoring, tuning, and adaptation to maintain effectiveness. Collect customer and employee feedback as a practice for continuous AI algorithm improvement.
As interactions increase, the model will automatically learn based on fresh data and improve.
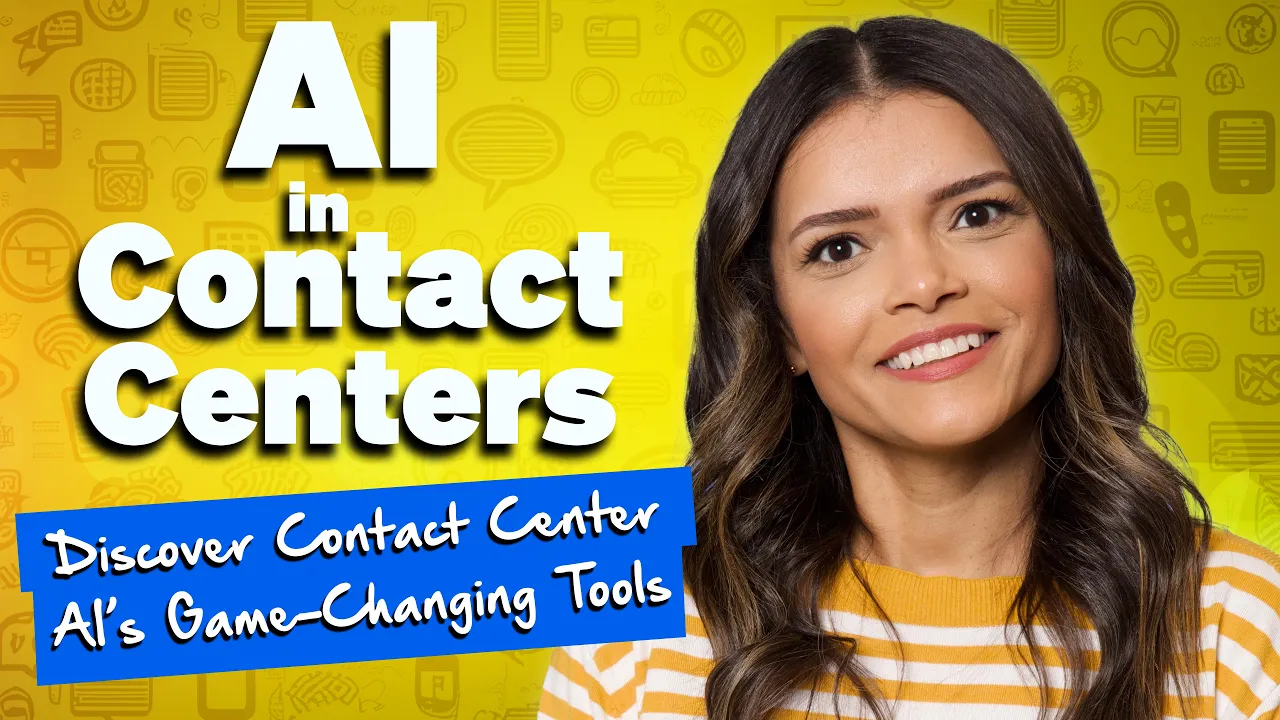
Examples of AI Agents in Contact Centers
In modern contact centers, AI agents are standard on the customer support front. These agents cater to different responsibilities, including:
- First-contact resolution: When a customer asks a billing-related question, the agent understands and looks into the customer’s account balance to suggest the best possible payment options. The agent goes beyond to offer discounts based on customer history.
- AI-powered retention agent: It looks for signals that tell whether a customer is about to churn. Then, it proactively contacts the customer to offer loyalty perks and special discounts.
- AI sales assistants for call centers: These AI agents listen to calls to detect objections and offer real-time suggestions. They automate the administrative workload and repetitive tasks, such as summarizing calls and updating CRM, making agents more effective in actual selling.
- Multi-channel AI virtual assistant: AI agents let buyers use any channel, like chat, email, or voice, to answer questions that buyers ask frequently. The agent goes beyond to schedule appointments and process any related refunds.
Nextiva Is Leading the Future of Contact Center AI
Nexitva’s AI-powered contact center solutions are positioned appropriately to integrate agentic AI with business systems. They unify channels like chat, phone, and email to deliver seamless customer support, regardless of the communication channel. The integration with CRM delivers the insights necessary to analyze buyer behavior. This supplies ample data to agentic AI, which is required to ensure accuracy.
Nextiva comes with intelligent automation, which is handy for increasing agent productivity and efficiency. When all of these are bundled together, it provides a complete package for integrating agentic AI with its contact center solution.
Onboard Nextiva. Get ready to equip your customer support team with the capabilities of agentic AI soon.
Top AI-Powered Contact Center Solution
Transform your customer interactions with a contact center platform that saves you time and money, reduces agent and supervisor stress, and flexibly adapts to fit your needs.
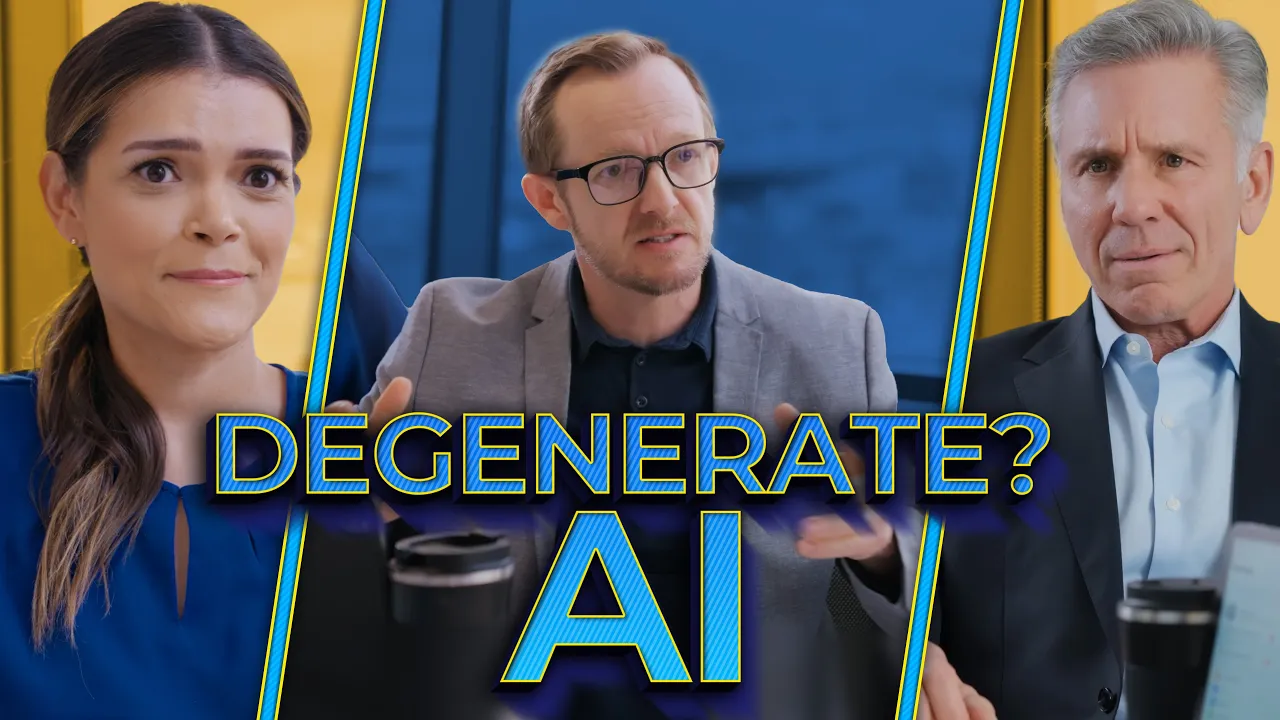
Agentic AI FAQs
Below are a few questions that raise readers’ curiosity about agentic AI.
There’s a difference in purpose and capabilities between the two. Generative AI creates content by learning patterns based on existing data and processing it in large language models (LLMs). It uses natural language processing (NLP) to understand the query and leverage machine learning (ML) to improve with subsequent interactions. It’s largely reactive and usually needs a prompt from the user to produce outputs.
On the other hand, agentic AI senses the environment, makes decisions, and performs actions to achieve its specific goals autonomously. It proactively takes initiative and performs complex tasks based on its planning and perception. Agentic AI continuously interacts with the environment and adjusts its behavior based on the feedback it receives.
Agentic AI can be used across many industries and scenarios. It would fit easily into customer service, sales, marketing, business operations, and software development. Consider these areas with a focused objective, a reliable dataset, and flexibility or creativity in the outcome.
If agentic AI is trained on biased data, it might make decisions that are biased and ethically questionable. At times, it can be tricky to understand or explain complex AI decisions, which can create problems if it doesn’t work as expected. These agents make decisions independently, which may not always be what users anticipated.